In the pursuit of a sustainable lifestyle, many individuals adopt eco-friendly practices such as recycling, energy-efficient appliances, and alternative transportation. However, a recent study conducted by researchers at Carnegie Mellon University and Hugging Face reveals that even the seemingly innocuous act of tapping into machine learning models for AI image generation can contribute significantly to one’s carbon footprint. This revelation challenges the common misconception that artificial intelligence exists in a virtual realm without tangible environmental consequences.
The study, touted as the first systematic comparison of costs associated with machine learning models, highlights the energy-intensive nature of AI image generation. The researchers discovered that generating an image using AI models consumes an amount of energy equivalent to charging a smartphone. The implications are alarming, considering the widespread adoption of machine learning models by over 10 million users daily.
The research team tested 30 datasets across 88 models, examining 13 different tasks, including image generation, text generation, image captioning, and summarization. Notably, the study found significant differences in energy usage between various types of tasks. The greatest energy expenditure was observed in Stability AI’s Stable Diffusion XL, an image generator, which produced nearly 1,600 grams of carbon dioxide per session—equivalent to driving four miles in a gas-powered car.
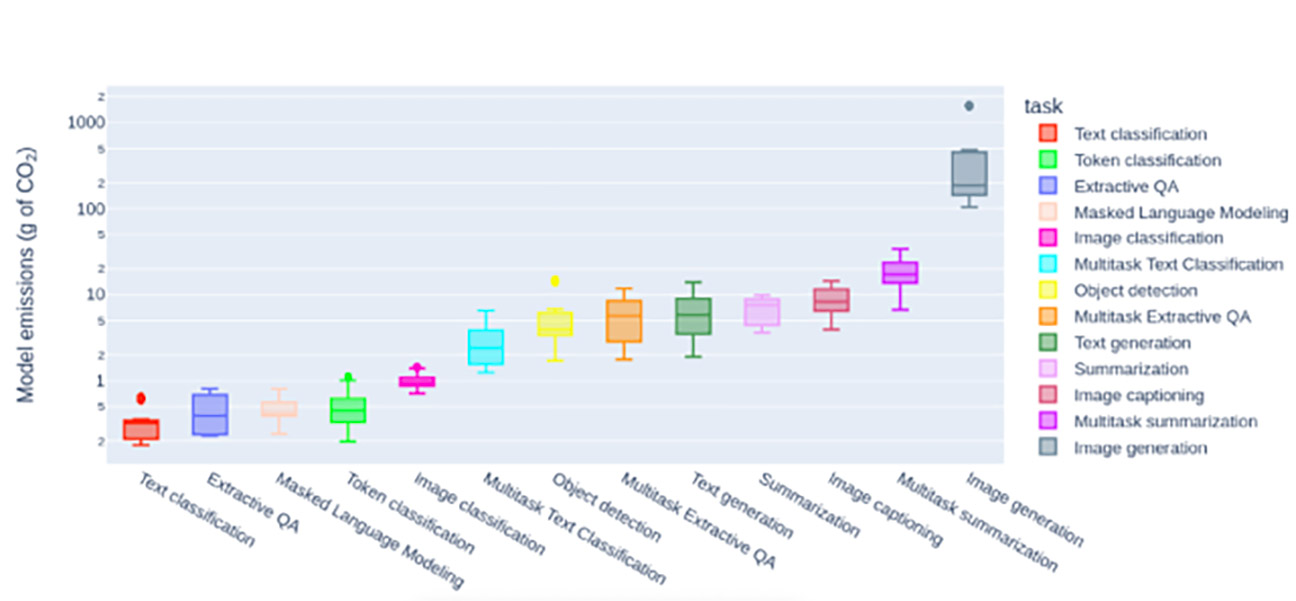
The study differentiates between generative tasks, which involve creating new content like images and summarizations, and discriminative tasks, such as ranking movies. It reveals that generative tasks are more energy- and carbon-intensive. Additionally, the researchers noted that using multi-purpose models for discriminative tasks is more energy-intensive than employing task-specific models for the same functions.
The researchers underscore the paradigm shift in AI model usage, moving away from smaller task-specific models towards multi-purpose models capable of handling a multitude of tasks simultaneously. They caution against unnecessary use of large models for specific applications, emphasizing the need for a conscious assessment of the cost and benefits of generative AI.
While the carbon dioxide emissions for individual AI tasks may seem negligible, the cumulative impact becomes substantial when multiplied by the millions of users relying on AI-generated programs daily. This raises concerns about the collective environmental impact of AI image generation and its potential hindrance to global efforts to reduce environmental waste.
The study serves as a wake-up call, urging individuals and industries to consider the environmental costs associated with AI image generation. As technology continues to advance, it is crucial to strike a balance between innovation and environmental responsibility. Conscious decision-making, judicious model selection, and a shift towards sustainable AI practices are essential for mitigating the carbon footprint of artificial intelligence in our quest for a greener future.